Managing risk and change is difficult at the best of times. Many businesses use AI to make decisions which generate value but they are quite rightly keen to know if the models driving the AI are still supporting good decisions. The desire for certainty is understandable and we’ve been keen to reassure customers that even in the current unstable environment, this is arguably one of the few business risks that you can still control — the art of data science can help.
As you’d expect, we’ve had many discussions with data scientists as they endeavour to ensure that their models adapt and continue to be relevant. Regardless of the industries in which they work, there are some issues that apply for many organisations so we’ve been working hard to share best practices to help fellow data scientists address concerns and solve problems where we can.
The obvious question we’ve been tasked with answering is: ‘Which AI use cases are sensitive to extreme events?’ The answer is that, in general, AIs that describe systems that humans interact with have been much more sensitive to the recent extreme events. “Human interaction” needs to be defined quite widely, though. For instance, changes to a production line’s operational parameters would qualify if they’re due to shifts in demand.
Customers subsequently want to know ‘How does this manifest itself? For some models, of course, the underlying behaviour being modelled (the “system”) has changed. Consider a retailer which has disinfectants and soaps in its product lines — the demand dynamics for these goods have changed dramatically and consumers are likely to be much less discriminatory in their buying decisions. In such cases, your production models may be describing behaviours that no longer exist.
In other cases, the system hasn’t changed, but some of the data is reaching new peaks or troughs that didn’t occur in the machine learning model’s training data. Some machine learning algorithms (such as decision trees and their relatives) can’t extrapolate; neural networks and linear models are good potential replacements here, although they should still be stress-tested to check that their extrapolations make business sense.
Coronavirus Diary: the impact on senior level recruitment in the technology industry
How can these changes be detected and measured?
Given the current crisis, well-designed governance and monitoring processes for deployed machine learning models have become even more important. A central registry of deployments is crucial and should include a dashboard showing the service health, data drift, and accuracy of each deployed model.
Capturing information on prediction accuracy is ideal but not always available quickly: for instance, if you’re predicting whether a customer will churn in the next six months, or will default on a five-year loan. In these cases, it’s particularly important to track how similar or different the data being scored is in comparison to the original training data. Model sensitivity to changes in data can be understood using partial dependence analysis — measuring the sensitivity of model outputs to changes in one or more inputs, other things equal. Stress tests simulate extreme readings by adapting existing training data and compare resulting predictions with those made on the original data.
Customers want to know ‘What can be done to address them once detected?’ This depends very much on the answers to the previous questions. If the machine learning model is making high-volume predictions where outcomes are known quickly, you should retrain the model frequently, or at least determine an accuracy threshold under which to trigger re-training. Training a model entirely on Corona-era data and comparing it to existing production models can also give useful insights into whether and how the behaviours described are shifting.
Lower-frequency models will also benefit from updating with more recent training data; should model accuracy fall as a result, this is another indicator that the system dynamics are changing. Falling accuracy can also be addressed by re-framing the models. For instance, if it’s becoming harder to predict how profitable an individual customer will be, it may be easier to predict whether profitability will exceed a certain threshold. If there just isn’t the data to update production models isn’t viable, consider how you can change the way you use your existing machine learning models. This may involve adjusting some data inputs, or indeed adding a penalty to some or all of the model’s predictions. The exact shape of these adjustments will need expert judgment, sometimes informed by whatever history is available.
Finally, of course, people have wanted to know ‘Is this really my problem?’ An informal survey that we conducted in early April indicated that over half our customers have already seen noticeable changes in the data flowing into their models. As the economic consequences of the pandemic become ever more pronounced, it’s well worth making sure that you are prepared. Good AI governance is therefore a necessary condition for weathering this particular storm.
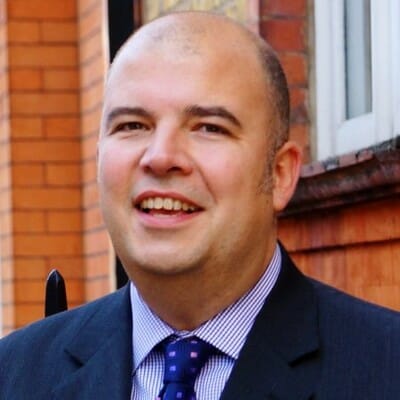