For many years, online advertisers have been using cookies to track website visitors, improve the user experience, and collect data to target ads to the right audiences. Marketers value what cookies can tell them about what visitors are doing online when they aren’t on their website. This matters, as Amazon has taught us the value of being able to predict what customers want to buy by analysing online sales data. It’s a technique all modern brands are eager to apply. Today’s digital consumers need intelligent, highly context-sensitive recommendations and prompts. “You may also like” is a phrase that ushered in a new era in customer relations and tailored suggestions. As a result, brands can maximise the value they deliver, secure customer loyalty, and cross- and up-sell.
There’s one small problem — third-party cookies are on their way out. This highly useful functionality is set to end, with Google‘s efforts to phase out the third-party cookie on Chrome browsers by 2022. There’s no need to be unduly worried, however. Graph database technology is being used to construct recommendation engines that don’t rely on third party cookies. These recommendation engines can make use of web logs to offer the same Amazon-level of personalisation advertisers are used to — in fact, arguably more.
Is graph technology the fuel that’s missing for data-based government?
A proven technology for enabling real-time recommendations
Graph databases cannot be simply exchanged for cookies. But they’re another route to the same thing: knowing your customer. We want to be intimately familiar with customers’ buying history to analyse their current choices before matching them to the most appropriate products. We must do all of this analysis in real-time before the customer moves to a competitor’s website, and a proven technology for enabling these real-time recommendations is graph technology.
Graph technology easily outperforms relational and NoSQL when connecting masses of buyer and product data to gain insight into customer needs and product trends. For example, eBay uses graph databases for a real-time recommendation engine. In the words of senior developer Volker Pacher, “we found [graph technology] to be literally thousands of times faster than our prior MySQL solution, with queries that require 10-100 times less code. Graph provides eBay with functionality that was previously impossible.”
To make real-time recommendations also requires the ability to instantly capture any new interests shown in the customer’s current visit. Matching historical and session data like this is easily achieved with a graph database. As a software developer at a leading big box retailer said, “graph helps us to understand our online shoppers’ behaviour and the relationship between our customers and products, providing a perfect tool for real-time product recommendations.”
Moving beyond third party cookies
And as we head toward a post-cookie future, retail and media firms can now analyse their weblogs to find connections over time. By analysing web traffic and click stream data, marketers can extract unique individual profiles. Identifying – and de-anonymising- users builds the foundation for more accurate recommendations, which result in a higher average number of visits per profile and creating better models of consumer habits. In the US, for instance, Meredith Corporation, a $3 billion media conglomerate with over 30 top consumer brands and a digital presence that reaches more than 180 million users a month, is using cookie co-occurrence, rather than third-party tracking data, to build unique customer profiles. Its senior data scientist, Ben Squire, said: “With millions of views and millions of unique visits per month across different topics and lifestyles, our consumers trust us for information on things that affect their daily lives, as well as pique their interest. By understanding and analysing this content and how it’s consumed, we strive to serve the needs of our audiences and advertisers alike.”
Historically, Meredith identified anonymous users through third-party cookies, but cookie loss across diverse devices and ITP 2.3 browsers that block cookies by default increase the difficulty of relying on them, which led to inefficiency. “If the cookie ID used in the models doesn’t appear again, then the money, time and effort that goes into building those models is lost,” he said. “Knowing your audience is not good enough; you need to see them again in order to act upon it.”
Meredith changed its approach. The company’s rich mix of media content naturally generates multiple, disparate streams of data. Meredith data scientists blended that data to find ways to identify users across those streams, and something interesting jumped out — pattern matching, which showed that the cookies designed to identify unique users were repeated across different data streams.
Looking beyond digital marketing
‘A high-definition view’
As a result, the company built an Identity Graph incorporating more than 20 months of user data from first- and third-party sources. The graph database has more than 4.4 terabytes of data across 30 billion nodes, 67 billion properties, and 35 billion relationships. 350 million profiles that would have been considered unique individuals with different interests and patterns have been consolidated into 163 million richer and more accurate profiles. A high-definition view of user interests and preferences fuels stronger models, which leads to more relevant content and more users returning over time.
The company used graph algorithms to transform billions of page views into millions of pseudonymous identifiers with rich browsing profiles. The move has completely overhauled its understanding of customer behaviour. “We have increased our understanding of a customer by 20 to 30% by looking at how the data connects over time, rather than just looking at individual cookies themselves,” Squire confirmed. “Instead of ‘advertising in the dark,’ we now better understand our customers, which translates into significant revenue gains and better-served consumers.”
There are significant learnings here for other advertisers looking to survive the end of the third-party cookie. To wield the powerful recommendations engines brands need to compete with Amazon and other born-digital brands, leveraging data connections and joining the dots between the relationships has to happen. This is where graph software can help firms, both with your next marketing and advertising offer. And perhaps, your long-term survival in our looming cookie-less age.
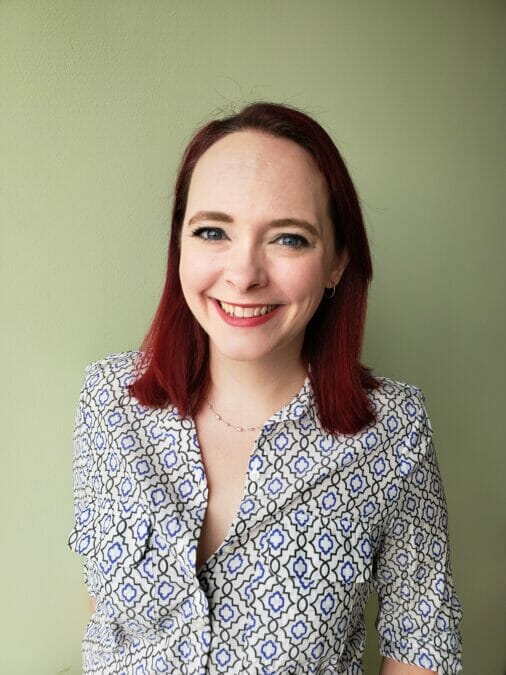