While RPA is geared more toward backend automation, conversational AI lends itself to automating human-facing and more personalized interactions. In some use cases, the two technologies intersect. But where does backend process automation meet conversation-driven automation?
Spot the difference
RPA involves the management of rigid, predictable processes, where there is no ambiguity about the request, which contrasts with conversational AI, where user intent dictates which tasks are undertaken in response to a request. Chatbots or digital assistants use messaging interfaces, or voice control, to have a conversational interaction. The fluidity of conversation requires natural language processing (NLP) automation to be highly flexible, so that the intent is correctly interpreted and the right tasks are undertaken to resolve the request.
Supporting customer journeys
Wherever a process involves human conversation, such as customer or HR interactions, conversational AI comes into play.
Consider the scenario of purchasing a new insurance policy. Usually, insurance agents go back and forth with a customer to gather multiple proof documents that need to be validated and attached to the customer record so that the policy can be finalised. Dealing with large numbers of new customers and managing their onboarding is time-consuming and costly for insurance companies.
Using a chatbot or digital assistant to ask “Please upload an image of your driver’s license here,” or, “Can you upload a copy of your recent banking statement?” takes the onus off the human agent to keep chasing down these documents, and also offers a more seamless and convenient customer experience as they go through the onboarding journey. This is the essence of conversational AI; it improves digital customer engagement and service outcomes, while reducing the cost of managing routine steps in a user journey.
On the backend, RPA can come into play in the onboarding scenario, automating repetitive compliance checks on gathered documents and updating systems of record with the customer information. The two together can be very powerful in making complete customer journeys seamless, faster and more efficient.
Gartner: 5 actions to help customers through the Covid-19 crisis
Complementary technologies
Conversational AI now allows businesses to automate key interactions with customers and/or employees, enabling a whole new wave of automation potential that, combined with RPA, can significantly reduce the need for human interventions in end-to-end business processes.
RPA and conversational AI can work hand in hand. For example, when a customer applies for a mortgage there are many steps they have to go through, which introduces friction and inefficiency that can result in the bank losing the customer.
A mortgage bot that can engage with the customer right at the start of the application, request their proof of ID, earnings, and recent utility bills and then pass this to back office processes for validation, removes much of this friction. RPA can be used for the validation processes, while the digital assistant manages questions from the customer, understands intents, gathers the relevant documents and keeps the customer informed of any issues. The property valuation can be automatically provided via RPA using property market data, so that the customer can be proactively updated on the status of their mortgage application via the digital assistant.
Who’s in charge?
RPA initiatives are generally led by IT, with input from business departments such as finance, production, or distribution, and have the primary goal of reducing cost and increasing efficiency by reducing manual processes and minimising human involvement.
Conversational AI is designed to improve and automate engagement and reduce cost, while still enabling bots to handover to humans when required. As such, it is normally led by business departments such as customer service, HR, and sales, with limited input from IT.
How UK retailers are using AI to enhance safety and operations
AI without data scientists?
Many organisations believe that artificial Intelligence is complex and costly, requiring large budgets and teams of data scientists to build the natural language models and machine learning algorithms. However, building conversational AI bots doesn’t necessarily require this level of investment. Natural Language Processing (NLP) engines such as Google DialogFlow, Amazon Lex, and Microsoft LUIS are widely available and make it easy enough to populate a bot with intents and utterances that are at the heart of designing a conversational experience that can be used to automate interactions.
Low-code
In addition, conversational AI platforms have emerged that offer a low-code approach to building chatbots, creating workflows, and integrating securely to common business systems so that business people can design and deploy their own digital assistants without needing coding or AI skills. Out-of-the-box bot blueprints and tools help businesses get chatbots to market faster, with less reliance on IT resources or data scientists, and with the ease of clicking, dragging and dropping.
How low-code can help to overcome the AI skills shortage
Conclusion
Enterprises are working to digitally transform core business processes to enable greater automation of backend processes and to encourage more seamless customer experiences and self-service at the frontend. We are seeing banks, insurers, retailers, energy providers and telcos working to develop their own digital assistants with a growing number of skills, while still providing a consistent brand experience.
Developing bots doesn’t have to be complex. It is more important to carefully identify the right use cases where these technologies will deliver clear ROI with the least amount of effort.
Whether an enterprise is applying RPA or conversational AI, or both, it’s important to first understand the business problem that needs to be solved, and then identify where bots will make an immediate difference. Then consider the investment required, barriers to successful implementation, and the expected business outcomes. It’s better to start small with a narrowly focused use case and achievable KPIs, rather than trying to do too much at once.
Conversational AI and RPA are very powerful automation technologies. When designed well, a chatbot can automate up to 80% of routine queries that come into a customer service centre or IT helpdesk, saving an organisation time and money and enabling it to scale its operations. However, outliers or specialist cases are still better handled by human agents. The Pareto Principle also applies to RPA. Automation at its best takes care of the majority of the routine and repetitive tasks, leaving the more unique, valuable, and rewarding work for humans.
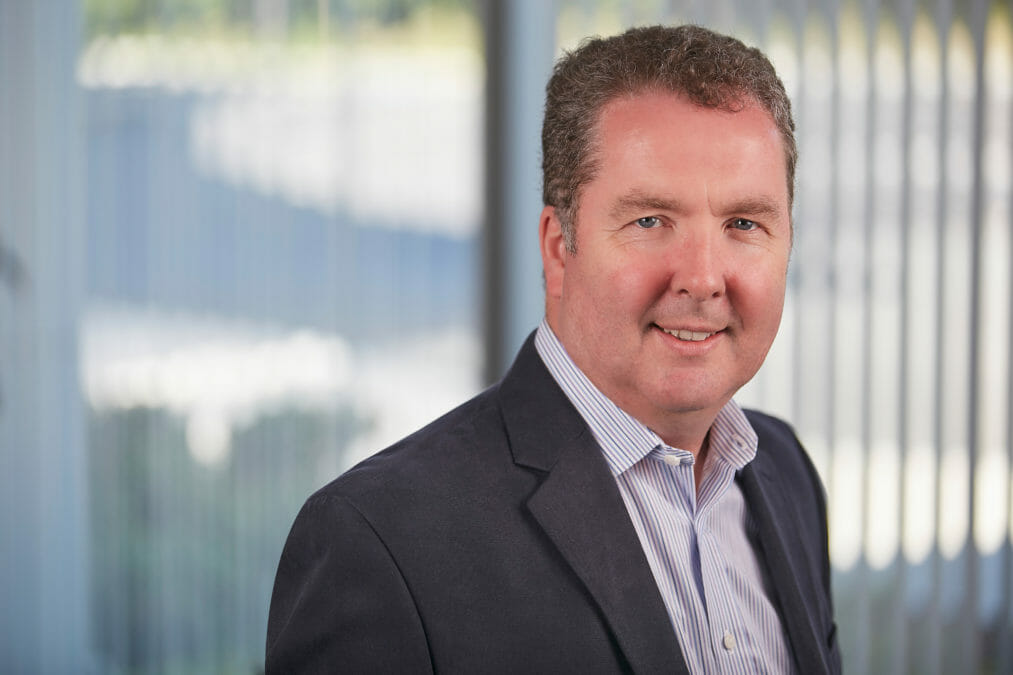