Although deemed by some in IT as outdated, the mainframe is still going strong in major large organisations. They are often responsible for the safe, secure and reliable running of mission critical applications, such as enterprise resource planning, (ERP), global point of sale (POS) or stock and inventory systems.
Mainframes can handle huge volumes of data incredibly quickly, and due to the nature of their internal programming, offer a high level of data integrity. They are also extremely powerful, often with extensive processing capability and massive storage arrays (the Latest IBM Z14 has 100’s of processors and 32TB of RAM for example).
For a number of years, they have also been able to run the Linux operating system (whereas previously they were often running proprietary operating systems), which has opened the doors to a number of new applications and capabilities previously not available such as analytics, artificial intelligence and machine learning
So far, so good for analytics, AI and ML.
The mainframe skills crisis forcing the move to cloud deployment models
When we look at delivering/building any application to deliver analytics, we are totally reliant on the quality and integrity of the data. If the data isn’t right, or indeed, has been aggregated into another platform such as a data warehouse or dumped into a data lake, the end user isn’t getting the full picture of what is going on. By looking at granular-level data, close to the source, we can be sure that we are seeing the real information that we need to make decisions.
When we move into the realms of AI and ML, the more data you can use to train and build models the more beneficial the outcome. Therefore, it seems to make sense to deliver these solutions on a mainframe; closer to the data and faster to analyse. It can also be harder to get data out of a mainframe into a secondary data analysis tier, as this results in a processing overhead (more on that later — quite often companies use the quieter times in the business to manage tasks like data extraction).
Running mission critical applications on the mainframe
But let us take a step back for one moment. We have already said that mainframes are responsible for the reliable running of mission critical applications. One of the downsides to a mainframe versus a cloud solution is that they have finite resources available to them (although this is a lot of resource in some cases), and if we start to put more pressure on the processing capability of the mainframe, then your mission critical applications could suffer.
One of the reasons for this is that AI and ML applications are very very hungry when it comes to performance and need lots of compute cycles to sift through data to create the models to deliver value. For best performance, AI and ML often require specialist hardware such as graphical processing units (GPUs) or software such as Tensorflow or Caffe, which are not often found in mainframes or skills found in those supporting mainframes — and they can be very expensive to hire!
Two-platform IT: Why the mainframe still has its place in the modern enterprise
It need not be an either/or for cloud and mainframe to drive digital innovation. Read here
One other thing to remember is that AI and ML models work best with a targeted approach to data – we don’t just want to look at all the data we have, as that would require supercomputers far more powerful than a mainframe (there are many papers on this, but it increases in non-linear time dependent on the size of the data processed e.g. 100×100 matrix processes take n2 longer that a 10×10 matrix). There has to be some thought given to what data we need to look at.
One approach would be to run a simple scan over the data to look for patterns that may be interesting to an AI/ML programme, and export that smaller amount of data for modelling and training – this could be done outside the mainframe (this is a similar model to edge computing; but that is another story). Another may be to simply extract some defined ‘core data’ and explore it using data mining tools, prior to ingestion into a dedicated ML environment.
An alternative approach, and one that resonates with the world of ‘big data’, is to start with a small scale, low cost project first. Initial work on AI should be done at low cost and when (and if) it is showing benefits, it should be scaled to use the resources appropriate to the return that the AI is going to deliver. CTOs, therefore, should be considering small pilot projects using non-core resources and potentially with specialist consultants rather than expensive internal hires before fully committing to AI and ML.
So in conclusion, the modern mainframe is more than capable of running analytics, artificial intelligence and machine learning; but the question is; is it worth the risk, and at often great expense?
It may well be an option for those large organisations who are unwilling to move to a cloud offering, or have already invested in mainframes and the software that powers them, however, it looks like it could be a risky business to me.
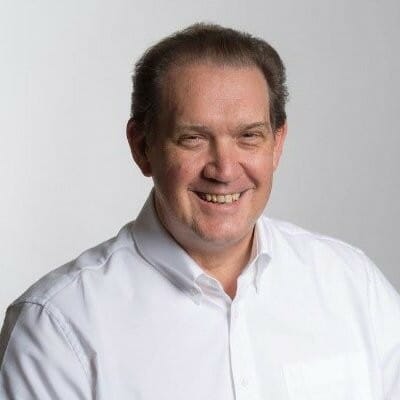