The use of deep learning in the cyber security space is an emerging trend. But, it has the potential to transform a security model that is currently broken, by predicting new attacks before they’ve breached an organisation’s network or device.
“Cyber security has a coronavirus situation every day,” said Jonathan Kaftzan, VP Marketing at Deep Instinct, during his presentation as part of the latest IT Press Tour.
Deep learning neural models can predict new variations of existing cyber attacks that occur daily, while the majority of current solutions in the market can only detect infected systems or anomalies, contain and remediate them — this is costly and unsustainable.
“Existing solutions need to wait for something to happen before reacting to it” – Kaftzan
Deep learning technology, a subset of machine learning algorithms (which is itself a subset of artificial intelligence algorithms), can predict and protect organisations from known and unknown cyber attacks in real-time, while mitigating the problem of false positives. It is changing how organisations build and manage their cyber security stack.
Many traditional solutions, as well, can only protect specific domains or operating systems (one vendor for Windows and Android, for example). A single platform that can handle any threat at any time is more viable.
Before delving into deep learning and cyber security, it’s important to identify why the cyber security model is broken.
Is the cyber security model broken?
From 2008 to 2018, the number of data breaches has doubled, from 636 to 1,244. Files and records exposed has also jumped from 35.7 million to 446.5 million over that same time period.
The problem is getting worse, despite investment in cyber security increasing by 30%. Gartner has predicted that the market will be worth $248.6 billion by 2023, according to Statista.
“There has been a huge growth in cyber security investment, but nothing has improved. In fact, it’s got worse. The cyber security model is not working,” stated Kaftzan.
Cyber security best practice: Definition, diversity, training, responsibility and technology
Why?
1. Volume — more than 350,000 new malicious programmes are created every day (mostly by machines). It is easy to modify existing malware and create a completely new cyber attack. It is overwhelming.
2. A question of when not if — 67% of CIOs thought the possibility of their company experiencing a data breach or cyber attack in 2018 was a given, according to a survey from the Ponemon Institute.
3. Cost — a big breach can cost an enterprise between $40-350 million.
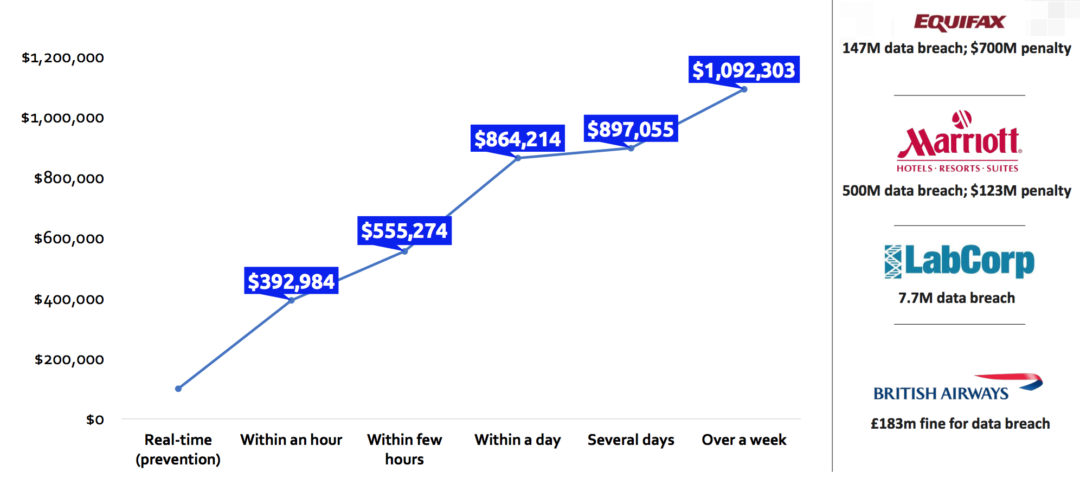
4. Skills shortage in cyber — 69% of organisations say their cyber security teams are understaffed, while there will be as many as 3.5 million unfilled positions in the industry by 2021.
5. Complexity of cyber attacks — the level of sophistication and complexity of cyber attacks is increasing. AI-based malware, and adversarial learning (using a neural network (DL or ML) to attack another neural network) is also beginning to threaten networks.
There have been 2x the number of data breaches and over 10x the amount of records exposed in 2018 compared to 2008
Deep learning in cyber security: is real-time threat detection and prevention possible?
By using a deep learning neural network algorithm, organisations can detect and prevent known and unknown cyber security threats in real-time.
Referring to Deep Instinct’s platform, Kaftzan said: “The time it takes us to analyse a file before you’ve even clicked it is 20 milliseconds. We’ve never seen it before to assess whether it is malicious or not. In another 50 milliseconds we’ll be able to tell you where the attack has come from and what it is autonomously, without any human being involved in the process. The time it takes to remediate and contain the attack is under a minute.”
SE Labs tested the solution and found it had a 100% prevention score with 0% false positives (when system flags a security vulnerability that you do not have).
HP is an investor and strategic partner of Deep Instinct. It has installed the technology in all the laptops they sell to the enterprise market — “millions of new laptops [HP Sure Sense powered by Deep Instinct] are protected using our technology,” added Kaftzan.
What is AI? A simple guide to machine learning, neural networks, deep learning and random forests!
The benefits of deep learning in cyber security
• Predictions of unknown threats.
• Zero time prediction and detection.
• Zero time classification.
• Works across any device, operating system or file.
• Doesn’t rely on connection (edge deployment).
Deep learning vs machine learning
Deep learning is a sub category in a family of algorithims under machine learning, while machine learning is a broad set of algorithms under artificial intelligence.
Everyone is talking about AI, but it has been around for many years. You can define the technology as a system that mimics human intelligence by making decisions. There are many forms of human intelligence, such as if you do a. then b. will happen — many systems are already using this type of rule-based decision-making.
“In this definition, using AI is the norm,” continued Kaftzan.
Machine learning was developed in the 1980s. Here, the algorithms could learn from datasets and make decisions based on that.
“Machine learning couldn’t improve human challenges until 10 years ago, when deep learning neural networks were introduced. They became available because of better infrastructure (GPUs),” explained Kaftzan.
Machine learning is reliant on the human stack. It limited, therefore, by the data (under 2% of data is analysed), the human (lack of knowledge and expertise), adversaries (mutating and growing cyber attacks) and size of the datasets.
Attackers can also hide the malicious features through things like encryption, commonly known as feature obfuscation.
An end-to-end deep learning framework, however, is the only algorithm in the AI family that can analyse and make assumptions from all the raw data without the involvement of the human.
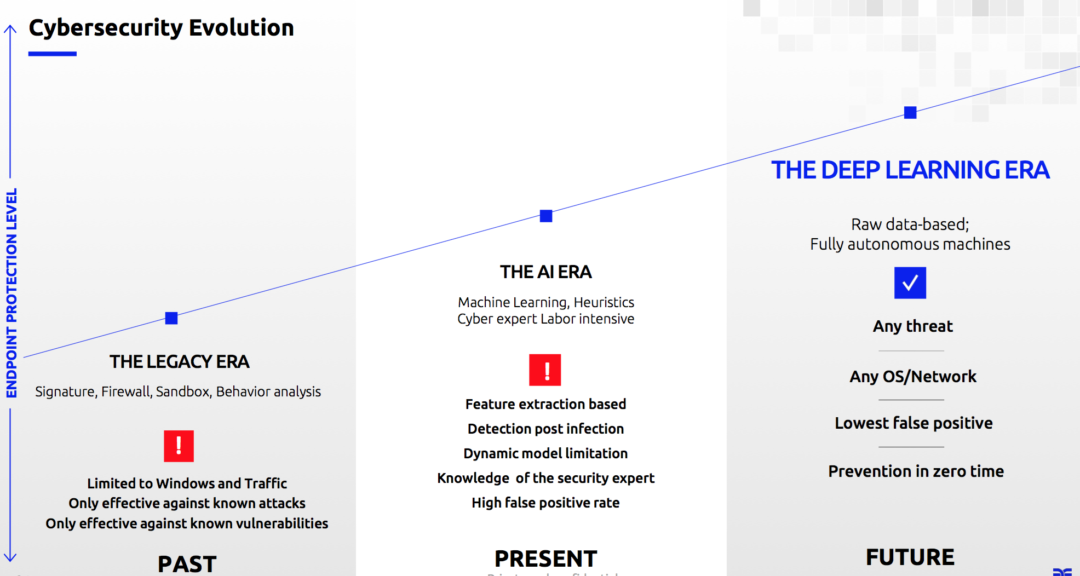
Growing prevalence of deep learning in real-world solutions
There is a growing prevalence of deep learning in real-world solutions, but the technology is lagging in cyber security:
• Computer vision: 98% deep learning, 2% traditional machine learning.
• Speech recognition: 80% deep learning, 20% traditional machine learning.
• Text understand: 65% deep learning, 35% traditional machine learning.
• Cyber security: 2% deep learning, 98% traditional machine learning.
End-to-end deep learning for cyber security
An end-to-to-end deep learning framework can predict the mutations of existing malware and prevent them in real-time, before the impact can be felt on a device or network. The algorithm is developed entirely on C/C++ and is optimised using NVIDIA’s GPU training (before it is deployed on endpoints using regular CPUs).
Deep Instinct is pioneering deep learning in the cyber security space. But, there are a number challenges. The main one is that there are not enough experts that can build deep learning algorithms — they’re all snapped up by the big companies, such as Baidu (speech recognition) and Google (NLP) after university.