The hope for artificial intelligence is that it will work for its users, not force its users to work around it. The progress to-date shows that AI systems are not exactly on the right path to fulfil that ambition.
There’s a belief that enabling AI systems to explain or justify their actions is equivalent to an ease-of-use. But ease-of-use, i.e. usability, isn’t just about transparency in decision making; good AI interaction design must consider the experience and aims of users and incorporate this into the development of AI systems.
The next stage of business change: Human-centred digital transformation
The development of search engines in the 1990s serves as a lesson for how people-centric design ambitions can fall by the wayside. Back then, individuals interacted with search engines as they would with each other; they would ask search for questions and state problems or desires, like learning about a news event or finding a vet. In time, people learned that this natural modality for interaction with a search engine yielded poor results, which encouraged us to shift to the keyword-based search patterns we use today (and spelled doom for Ask Jeeves).
To put it differently, the user-centric experiment failed: the interaction was designed around the computer rather than around the experience of the user and eventually, it was the user that changed, not the design. This pattern continues to pop up when we create new intelligence-powered tools; just think of how we first interacted with Siri on the phone.
This history provides us with some valuable lessons as AI systems become more commonplace. We’re at a critical juncture; over the next year, AI will become more accessible to those outside of major tech companies through the shift from recommender systems to reinforcement learning.
It’s unclear if these new systems are designed to fit the needs of its consumers. Without any intentional shifts, adaptation from human users will still be required – as of now, Accenture estimates that 67% of AI users must learn a new skill or interaction pattern to fit AI into their everyday life.
We all understand that the gulf between builders and users is a key reason for this. With most highly-trained AI scientists making the jump to either a large tech company or a vibrant startup after college, most companies – even major Fortune 500 firms – have to develop talent from within. Thankfully, the genesis of so-called ‘citizen data scientists,’ i.e. homegrown AI talent that comes from non-technology firms, may help to bring user-centric thinking into AI design.
The future of data scientists, automation and the citizen data scientist
These so-called ‘citizen data scientists’ think about AI differently — they know the business problem well, are fluent in the domain, and are used to understanding how to build solutions that prioritise solving business problems. In the early days, the feedback from people on the business side helped technologists simplify AI for use.
I think back a moment early in my career where a business engineer told me that the system I was building was technically fantastic, but the outputs needed to be created in a way that could be presented to metrics-focused executives. In response to this widespread issue, the concept of “answer-first” interfaces — where the UI is so simple that it could answer, even anticipate, all questions one could anticipate from the data — was born.
The shortcomings of this model, i.e. the potential for biases among data sets driving flawed outputs, have been widely documented. But it was a pivotal step forward for user-centric development. ‘Citizen data scientists’ were born from this dilemma and it’s their desire to solve business issues that can push the boundaries of AI design.
Change is afoot. Earlier this year at the ACM conference on Intelligent User Interfaces in Los Angeles, conversations between the hundreds of participants centred on how to make AI systems more human-centered and fluid for users. Amidst the buzz was the launch of Stanford University’s new institute for human-centered intelligence. There was a feeling amongst us all in the user-oriented AI community that we had reached a real turning point: AI developers are waking up to the notion that the technology needs to be developed for users rather than data scientists.
The progress is promising, but we still have a way to go and a lot to learn. Research has shown that a continued focus on building AI systems around the ability to justify their actions will make them harder to use. Instead, there is something to be said about the ability to integrate AI intro any user’s actions — in other words, making the technology so attuned to the user’s behaviour that the user doesn’t even realise it’s there. We have an opportunity and an obligation to create ‘user-first’ AI interaction design. If we succeed, we’ll all be “citizen data scientists” without even realising it.
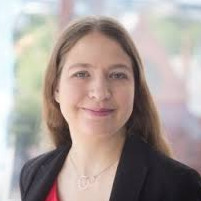