We asked 100 senior tech executives — CIOs, CTOs, and chief data officers — what they need to bridge data silos, boost AI/ML projects, and open up new revenue streams. A massive 88% said the same thing: knowledge graphs. Given that these executives represent large organisations across verticals using graph technology for a wide array of use cases, something’s clearly going on. So why is the knowledge graph —defined by Stanford University as “a compelling abstraction for organising world’s structured knowledge over the internet, and a way to integrate information extracted from multiple data sources” — becoming such a hot topic?
Leaders know the value of their data, keenly aware that it holds the answers to their most pressing business questions. They need insights to improve decision making and enhance business performance. Knowledge graphs can help these leaders because they function as a special non-disruptive insight layer on top of their existing data landscape. Knowledge graphs drive intelligence into data to significantly enhance its value — without changing any of the existing data infrastructure.
Another key driver that explains why business leaders are turning to this technology, is that knowledge graphs make incumbent technologies better. In the 1990s, there was a well-known BASF advertisement that said, “We don’t make a lot of the products you buy. We make a lot of the products you buy better”. Knowledge graphs act in the same way. They make existing technologies better by providing better data management, better predictions, and better innovation, in part because they fuel AI and machine learning. And importantly, knowledge graphs help make products better because they lend themselves to multiple use cases.
Could graph technology neatly fill the cookie gap for marketers?
The ‘X 360’ look
Let’s break knowledge graph use cases into two groupings: data management and data analytics. The data management knowledge graph’s aim is to drive action by providing assurance or insight. Data assurance knowledge graphs focus on data aggregation, validation, and governance, and include examples like data lineage, data provenance, data governance, compliance, and risk management.
Funnelling all your data into a knowledge graph can be a challenge. MANTA, built on a knowledge graph, is an automated lineage platform that shows users how data flows and transforms in its journey across systems. By embedding a graph database, customers quickly acquire a more holistic and scalable view of their data pipelines, allowing better governance, compliance, migration, and metadata activation.
By contrast, the data insight knowledge graph goes beyond visibility of information, and focuses on exploration, deduction, and inference of new knowledge. Examples include ‘Customer 360’, ‘Patient 360’, ‘Product 360’, ‘Agent 360’, and so on. In this category, there is also identity and access management, AML (anti-money laundering), root cause analysis, recommendations, and many others.
A standout example is a knowledge graph that tracks objects in space, both functional equipment and broken equipment, known as space junk. Called ASTRIAGraph, the knowledge graph aims to help monitor space objects in Low Earth Orbit for the purpose of long-term safety, security, and space travel sustainability. By utilising a knowledge graph, the ASTRIAGraph team relates and categorises huge amounts of disparate space domain data and tracks objects from mobile phones to massive satellites. The knowledge graph is used to predict the objects’ trajectory and assess risk. ASTRIAGraph provides a clear picture of a hugely complex bit of reality.
AI, machine learning, IoT and digital twin technologies
The magic of knowledge graphs comes into play as you use AI and machine learning to analyse them as a whole, uncovering patterns and anomalies. That’s where data analytics knowledge graphs come in. They improve decisions, forecasts, and predictions and prescribe optimal actions. Use them to predict churn and perform any type of what-if analysis.
Consider the power of such knowledge graphs in medical research. The German Center for Diabetes Research (DZD) is a case in point, as it accumulates a huge amount of biomedical data that is unstructured, heterogenous, and unconnected. The DZD set out to better understand the causes and prevention of diabetes. DZDConnect, its knowledge graph, gives more than 450 scientists fast access to all relevant information. The knowledge graph helps them to ask rich, complex questions as they seek to understand treatments and lifestyle interventions for this metabolic disease and its long-term complications.
Knowledge graphs absorb more and more data, while at the same time making that data more and more useful. DZDConnect was first built in 2017, starting with the DZD’s own clinical trial data and enriching it with large volumes of cross-disciplinary research data for conditions like stroke, heart attack, and cancer. Most recently, DZD expanded its knowledge graph with published medical research, adding in 30 million articles from PubMed. The DZD’s knowledge graph now has nearly 1.8 billion nodes and 4.9 billion relationships.
Another example is TCS IP2, an intelligent power plant solution built on a knowledge graph. TCS IP2 draws on the power of knowledge graphs for real-time power plant diagnostics and optimisation. Using a combination of AI, machine learning, IoT, and digital twin technologies in conjunction with knowledge graphs, the system can pre-empt failures, optimise operations, lower fuel consumption, cut emissions, and deliver other data-driven benefits.
What’s fascinating is that customers tell us their use cases start as data management knowledge graphs, but they often turn into data analytics knowledge graphs that support decisioning. Teams typically start with a data insight knowledge graph so that they can explore relationships, add graph algorithms to analyse network structures and discover patterns and anomalies, and use graph-native machine learning for prediction.
For machine learning, you need features, and features you extract using graph algorithms enhance your machine learning models to drive better predictions. More advanced graph-native machine learning learns from the graph to highlight missing data and relationships, making connections you don’t know are there and inferring data and relationships to add. With all of this, it’s not surprising that Gartner recently stated, “Up to 50% of Gartner inquiries on the topic of AI involve discussion of the use of graph technology.”
Summing up, you can see in a few steps how we’ve gone from data insight knowledge graphs to sophisticated knowledge graphs fuelling AI and machine learning. That’s a typical graph technology journey for our customers, with knowledge graphs at the centre. It’s a powerful trend that we see across every industry and in every data science team. No wonder the C-suite is waking up to the possibility of knowledge graphs. Should yours?
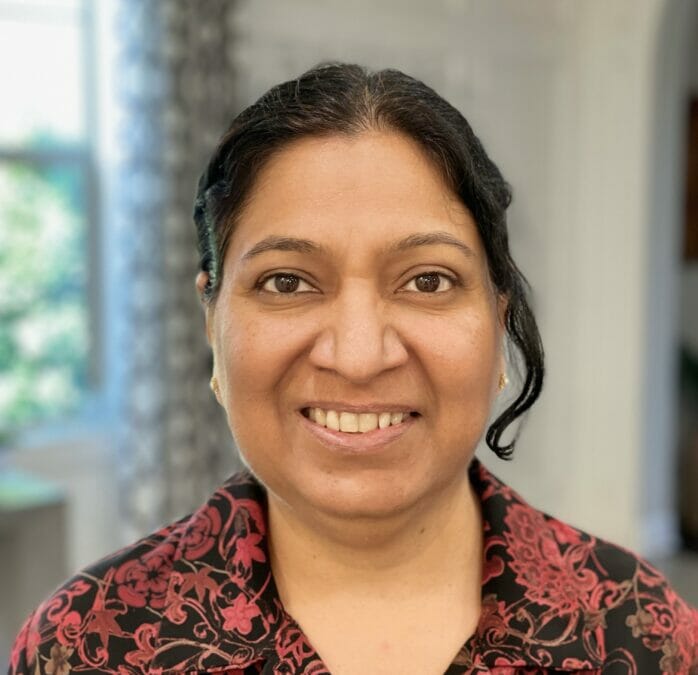