This article will explore the biggest barrier to AI adoption in healthcare.
Less than 4% of all physician-patient interactions involve AI today; by 2023, one in five interactions will include this strange technology.
If those statistics shock you, consider the end goals of most value-based healthcare programmes. Clinical department heads are under increased pressure to reduce costs, enhance the quality of care and improve patient experience — also known as the “triple aim initiative”. In an industry that’s known for moving at a snail’s pace, artificial intelligence is often viewed as healthcare’s magic bullet.
Artificial intelligence and the healthcare sector
Accenture predicts that clinical health AI applications could save the US healthcare economy $150 billion annually by 2026. It’s true that potential savings are enormous: In a 2018 survey of 15,000 US-based physicians, 56% cited “too many bureaucratic tasks” as the reason for their burnout. Since AI excels at automating rules-based, repetitive tasks, it could take reporting requirements from physicians’ workloads.
Outsourcing such bureaucratic tasks to AI would leave physicians more time to focus on increasing the quality of care, thus improving patient experience. But before you add machine learning to your clinical workflow, pause to reflect on the challenges involved.
AI technologies like machine learning allow software-based systems to analyse and find patterns within datasets. The size of your dataset is an advantage here, as machine learning needs positive and negative examples of specific classes.
To teach a machine learning algorithm what a heart is, you should expose the algorithm to images of a heart and images of other body parts (like knees and toes) so that it learns not to classify knees and toes as hearts. The more data within a dataset, the more likely you are to give your algorithm the training data it needs to find and correctly identify a heart.
AI and machine learning needed to improve the healthcare industry
At first glance, healthcare should have an advantage: This industry has a huge amount of data. In fact, the volume of healthcare data will surpass finance, media, and manufacturing via compound annual growth of 36% through 2025. This is due in large part to increased use of emerging technologies in healthcare, such as medical imaging, chatbots, and big data analytics tools.
Unfortunately, volume is just one aspect of data readiness: Quality is the other crucial component. And what healthcare data provides in volume, it lacks in quality.
In November 2018, the International Data Corporation (IDC) published a report assessing the “data-readiness” of four industries (including healthcare) on a scale of 1 (Critical) to 5 (Optimised). IDC ranked the healthcare sector at 2.4, reporting that 60% of survey respondents in the healthcare sector lack a data strategy.
GetApp’s own research echoes IDC’s findings. In April 2019, we surveyed nearly 500 small business leaders across five sectors to learn how they make decisions using data. Our respondents in the healthcare sector had the highest utilisation rate, meaning that they were least likely to have 10% or less of their data go unused:
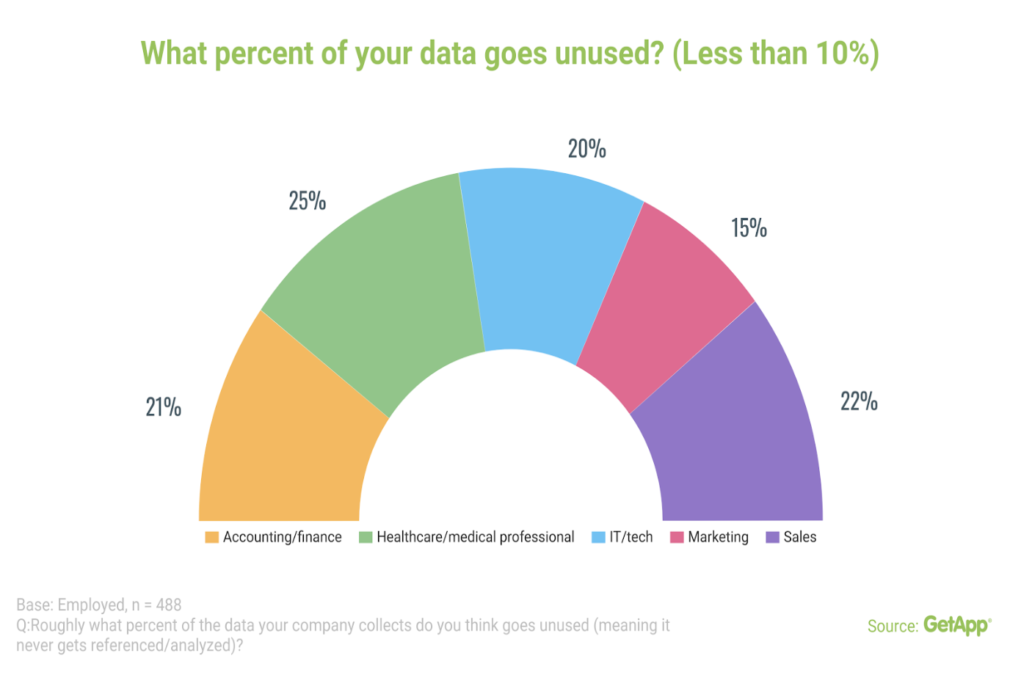
But when we asked respondents which aspect of the data analytics process makes them feel the least confident, 25% of respondents in healthcare said they felt the least confident in their ability to collect relevant data:
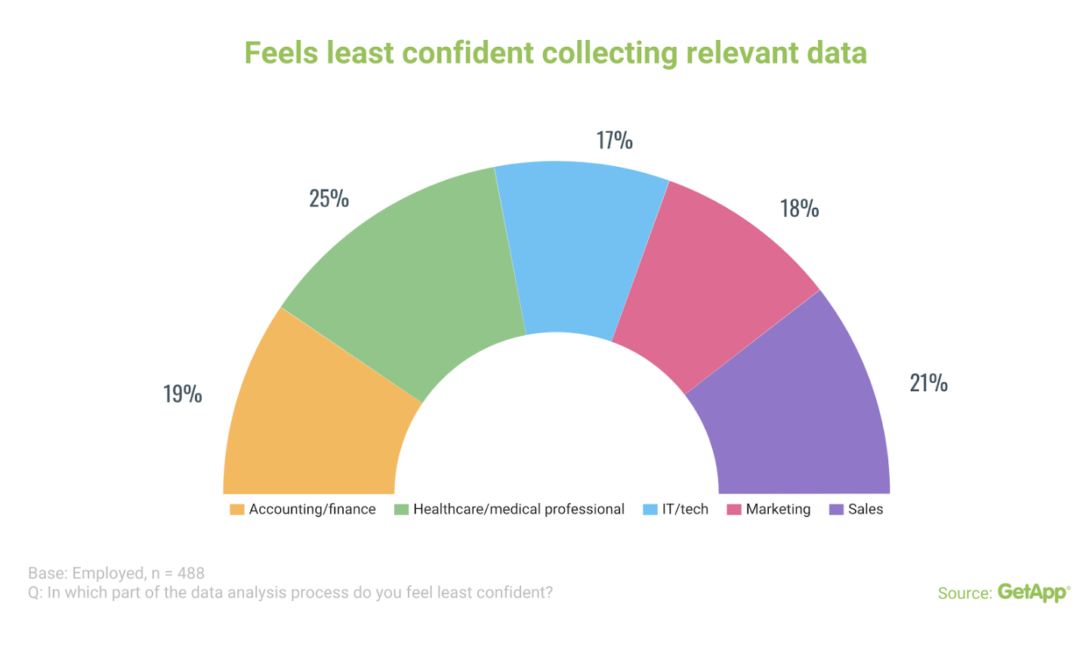
Regarding this question, respondents in healthcare displayed lower levels of confidence than their peers in accounting/finance, IT/tech, sales, and marketing.
In fact, 42% of respondents in healthcare also said they wanted more customer satisfaction scores before using data to make key decisions. And across all five sectors –including healthcare — data quality was their key concern.
Before the healthcare sector can adopt AI en masse, it must start by improving the quality and relevancy of the data it would use to train AI systems. Without a high amount of quality data to train such systems, there’s no hope of building AI into clinical workflows.
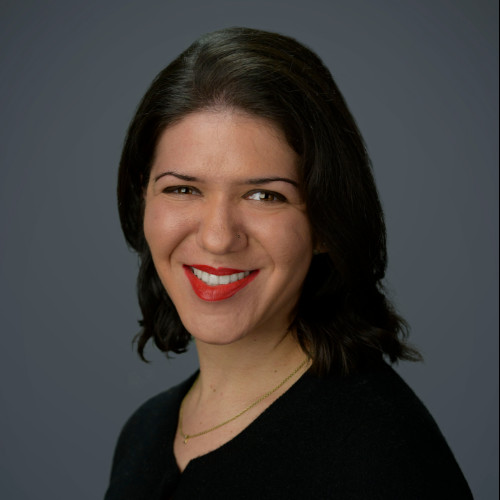