Infrastructure and network problems must be remedied at lightning speeds; ideally before the end user or customer even knows there is a problem. The accelerated digitisation of so many more parts of our economy and society gives incident management added urgency and relevancy.
Yet, even as they are more responsive to customer needs, modern applications involve rapid deployment of updates that place a strain on infrastructure reliability, triggering performance issues and even outages in digital services.
Having the correct tools to address incident responses is imperative to managing infrastructure reliability. Many of the more cloud-native approaches are too complex for site reliability engineers (SREs) and others to fully understand. They certainly need greater visibility, but also the capability to judge priorities and to identify and fix an issue swiftly.
This is where AIOPs is becoming a common approach, especially as the software and infrastructure estate that must be managed grows so rapidly and widely. AIOps gives teams the added security needed, by automatically detecting anomalies in their environments before they transform themselves into bigger, and more difficult issues to overcome.
Notably, AIOPs is even more effective as a site reliability engineering tool because of how the applications and infrastructure are mushrooming. It operates at its finest when large, expanding volumes of performance data are at its disposal. This data can include both observational and engagement data, as well as data from third-party tools. To assist teams in identifying, and diagnosing the problem, algorithms and machine learning tools are then applied across the data to increase intelligence about what is happening and helping to automate even more effectively how incidents are managed.
There are at least five ways that AIOPs are being applied in the real world:
1. Detecting incidents
This is the primary use case where AIOps is expanding the toolkit so a team can detect problems much sooner. AI and machine learning automatically begin to surface and understand anomalies, and then apply this learning to how systems and infrastructure are observed. What is being learnt here can drive a proactive approach that spots early warning signs and thus helps a team be aware of an issue before anything is noticed by a customer.
Training machine learning models to be future-ready
2. Reducing and cutting through the noise
Alert fatigue is a major problem in incident response. A barrage of alerts makes teams can become numb to all alerts, even if they are critical. Ideally, you need to suppress low priority alerts and group alerts that are related to each other. AIOps can correlate, suppress, and prioritise alerts, ending the misery of alert fatigue and enabling teams to double-down on the problems that are the most threatening to reliability.
3. Put it into context
Incidents are messy, fast-moving beasts. There is an overload of information that gets teams lost. They need a guide to give context and thus point them in the right direction. AIOps can do the job of mapping what is happening automatically and can deliver a holistic understanding of an incident. Context is invaluable to not only understanding but also resolving an incident.
4. Getting smarter and smarter
AIOps is a living, growing tool that is always improving. Past experiences, current usage, and user feedback create excellent data on which AIOps can train, helping identify and prevent issues similar to historic issues. With this continually growing wealth of information, models get smarter and deliver tailored correlations, insights, and recommendations.
UK’s leading AI startup and scaleup founders highlight the main pain points of running an AI business
5. Integrate data, integrate the team
Incident data from any source integrates with your current incident management tools and workflows. The more data you have coming in, the better trained your machine learning models will be, resulting in more tailored and useful results. An AIOps solution ingests data, enriches it with context, and sends notifications to the relevant teams or responders, all in the incident management tools teams are already using. This way, teams do not waste critical time switching between tools.
For organisations that have not yet started to apply AIOps, it may sound like a serious uphill job and, to be honest, there is a learning curve to ascend. Yet, there are some proven steps to starting AIOps.
First, consider what is the best use case or cases for you. Think small scale so you can learn, test, and grow from there.
Secondly, be transparent about what you are doing. People are resistant to change and you will need to put some effort in demystifying AIOps.
And finally, be ready for AI and ML to affect IT operations. The number of organisations relying on AIOps is growing and it is a technology that is going to become mainstream fast.
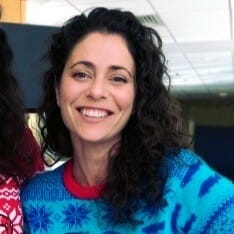