Donald Feinberg, vice-president and distinguished analyst in Gartner’s ITL Data and Analytics (D&A) group, explores the different kinds of cloud architecture for data management, and why D&A leaders need to balance the risks and benefits of each
The need for and use of data, be it customer or business data, is becoming increasingly advantageous for today’s organisations. It helps businesses stay competitive and ahead of the curve with intelligence to make smarter decisions, quickly.
However, it is important to recognise that a data-driven strategy can demand too much of a business – particularly if the right tools and solutions to manage those additional needs aren’t in place.
Solutions such as cloud data management architecture are critical, therefore. However, D&A leaders need to be aware of the different architecture choices – from on-premises to multi-cloud and intercloud. They need to understand the risks and benefits that come with managing data across diverse and distributed deployment environments.
Here, I take a look at the different cloud data management architectures and the considerations D&A leaders need to mindful of.
1. On-Premises to cloud
In an on-premises to cloud model (also known as “ground to cloud”), different components of an application architecture may reside on-premises and/or on one cloud. The database management systems (DBMS) might reside on-premises and the applications that connect to it may reside in the cloud — for example, a business intelligence (BI) dashboard application.
There are two variations of on-premises to cloud architectures:
- Active (formerly “architecture-spanning hybrid cloud”)
- On-demand (formerly “use-case-specific hybrid cloud”)
An active approach, as its name implies, deals with active data management between the two environments. This may include architectures with data residing both in the cloud and on-premises, such as the ability of the DBMS to have some replicas, partitions or shards residing on-premises and some in the cloud for the same database.
There are many application use cases for this kind of functionality, including: partitioning data by age, frequency of access or geography; dynamic capacity allocation to accommodate inconsistent, surge demand on resources; and regulatory requirements governing data locality.
In an active on-premises to cloud model, it is critical to understand the characteristics around the flow of data (for example, whether data is flowing into or out of the cloud and the expected volumes of data). There may be issues with latency — that is, the time it takes to move the data between on-premises and cloud. Additionally, there may be financial implications driven by CSP data egress charges. Integration, metadata and governance practices that span multiple environments must also be considered. Service level agreements (SLA) should be defined and tested. This may lead to a requirement of a special communications link between the on-premises and cloud components, leading to greater financial cost implications.
In an on-demand approach, components remain separate. Data is moved between environments only when necessary to support business activities like disaster recovery planning or development lifecycle functions. For example, any of the development, test, quality assurance (QA), disaster recovery (DR) or production instances of a DBMS may reside on-premises or in the cloud. Although financial and latency considerations remain important, compatibility is the primary concern in this scenario. Many organisations may not be comfortable with anything less than 100% code compatibility between the cloud and on-premises environments, in turn limiting the cloud service provider (CSP) selection to those that can meet these rigorous requirements.
Key considerations for on-premises to cloud deployments include: data movement in both volume and direction (active); and the compatibility of components between environments (on-demand).
Related: “On-prem first” – a hybrid solution for workflow storage — allowing organisations to adopt a hybrid approach, while centering application storage on-premise for security and reliability
2. Multi-cloud
Multi-cloud models incorporate one of more services from more than one cloud provider (and optionally may include on-premises or hybrid architectures). In this scenario, the difference is that services from multiple cloud providers are used. A DBMS offering and the applications that rely on it may be deployed both on-premises and/or on one or more clouds.
As such, all of the considerations of hybrid cloud may apply with the added considerations of deploying software in multiple cloud environments. These offerings have historically been limited to independent software vendors (ISVs) rather than native CSPs, as the ISVs have more of a vested interest in making sure that their software runs in as many environments as possible. However, cloud service providers are increasingly engaging in multi-cloud and intercloud scenarios.
The multi-cloud scenario generally appeals to end users who are concerned about cloud vendor lock-in and want to be able to move their applications easily to a different cloud provider. In providing a semantically compatible offering that runs identically in multiple clouds and on-premises, multi-cloud-capable DBMSs promise easier (albeit still not easy) migrations, as the primary concern will be migrating the data, not rewriting the applications.
If anything, for multi-cloud deployments, it is paramount for D&A leaders to consider the compatibility of components between environments and the different cloud capabilities for provisioning, management, and governance.
Related: Why businesses should embrace multi-cloud — Neil Templeton, vice-president, digital innovation marketing at Console Connect, discusses why businesses should embrace multi-cloud
3. Intercloud
Intercloud refers to managing data actively across more than one cloud. In an intercloud model, different components of an application architecture may reside on different cloud platforms and exchange data. For example, Microsoft’s PowerBI might connect to a Salesforce database that resides outside of the Azure cloud infrastructure.
Intercloud models are less commonly used today. At the same time, they are increasingly of interest to those seeking more advantageous pricing models, specific tools not available from other CSPs, risk mitigation through using multiple CSPs, and addressing data sovereignty requirements through diversified data location. For example, regulatory requirements might forbid data from residing outside a country’s geographic boundaries.
For intercloud deployments, D&A leaders need to be particularly mindful of data movement – both volume and direction.
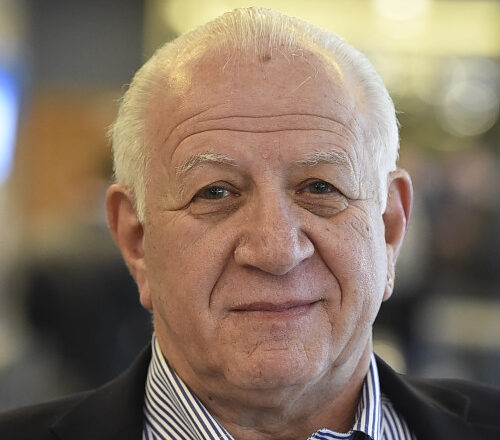