The Robotic Process Automation (RPA) market is booming, predicted to reach $4.4 billion in value by 2023. It provides businesses with a huge opportunity to automate manual, time-consuming, repetitive and transactional processes. RPA can help to improve process quality, speed and productivity and integrate legacy systems which is something that is increasingly important in the current climate as organisations seek to accelerate digital transformation projects.
It’s clear however that while RPA has the potential to be a very valuable tool, common obstacles to its success are business complexities, subjective decisions and unstructured data. RPA can only automate simple tasks. It needs processes to follow finite predefined rules with structured data.
The key to jump-starting digital optimisation projects is to connect the head (artificial intelligence and machine learning) with the hands (RPA). I’m talking about the convergence of RPA with AI and ML to create intelligent automation, which has the potential to massively increase the range of knowledge work that has previously been considered to be too complex to automate, and required human intervention to make predictions. With intelligent automation, AI and ML automates the decision-making and RPA automates the manual next steps within the process.
How RPA differs from conversational AI, and the benefits of both
How? At a high level, machine learning can be broken into two major components. The first part involves training models on historical data to make predictions. This involves collecting and preparing the data – often the most time-consuming step in machine learning – and concluding with a training data set that is labelled and ready for modelling. Next, models are built using algorithms for different types of data problems, i.e. classification, regression, binary. Once the model is built and deployed into production, the next component of machine learning begins – scoring unseen data against the built models. This is the step where RPA can ask the machine learning model what to do next, with the model providing a prediction decision for RPA to continue without human intervention.
IDC has identified digital transformation as a top trend in the life sciences and healthcare industry, so it’s no surprise that there is now increased interest from this industry in automation use cases where the addition of AI and ML with RPA could add value across the entire ecosystem. The aim is to create a scalable digital workforce that has the capacity to execute processes that don’t require human intervention, and deliver a return on investment in less than 12 months.
The key organisational benefit of using intelligent automation to remove human labour from mundane tasks in this instance is naturally enabling healthcare professionals to focus on higher-value, human-led decision making, diagnosis and treatment. Delivering a better patient experience and improving outcomes can be achieved by optimising patient engagement, providing clinicians with faster access to more information, which in turn enables them to provide targeted and tailored care.
Providing greater visibility of data in real-time is also being used by pharmaceutical companies and medical device manufacturers, for example, to eliminate potential compliance concerns by reducing fraud and error rates and to increase accuracy, safety and security. This is especially the case in the life sciences industry.
Intelligent automation is being harnessed to fast-track drug discovery, vaccine development, and clinical trials, by automating processes relating to documentation and regulatory monitoring. Removing bottlenecks is proving to be the key to addressing some of the challenges posed by the pandemic, especially with regard to providing test kits and Fast Track analysis.
AI use cases in healthcare for Covid-19 and beyond
The ability to standardise data, use larger data sets, remove biases, and train algorithms more efficiently to identify, for example, which compounds may be more effective or worth moving through the drug discovery process quicker, is providing results quicker and almost making it possible to do the work in advance. This in itself suggests that assessment, outcomes, the possibility of approval and efficacy could be done in the drug discovery stage, alongside clinical development, regulatory and document processing, potentially leading to virtual clinical trials.
Introducing more automation in laboratories will also enable data to be linked back into manufacturing, and other data lakes, to provide greater visibility of trends, faster and delivering scale manufacturing, and more agile supply chains which are major requirements, especially at this time.
Production demand forecasting is a core use case for example – predicting where there may be a surge of demand based on externalities such as a rise in the flu or increase in COVID-19, or a potential change in the population, may increase demand. Similarly, being able to monitor and track quality issues of pharmacovigilance and complaint handling – seeing trends regarding regulatory submissions or complaints as they come in, monitoring trends sooner, updating field teams so they can proactively manage issues (regarding samples and shipments for example) within days rather than weeks – can contribute to increased sales.
Fortunately, intelligent automation is enabling the life sciences and healthcare industry to manage and integrate legacy systems and achieve the benefits of digital transformation without updating software, developing APIs, or building a new system, within weeks, rather than months or in some cases years.
Key success factors behind intelligent automation
Data can be collected from multiple sources and must be cleansed and prepped before modelling is initiated. Instead of being locked in an ivory tower, AI and RPA are being democratised through intelligent automation. People can get direct access to data science and make use of the information themselves, instead of waiting to get that same information from a group that is siloed somewhere else.
Enabling the life sciences and healthcare industry to take advantage of these AI, ML and RPA tools and techniques to support AI-driven decision making and deliver ROI in a short period of time is increasingly becoming a practical reality.
The convergence of RPA and AI and ML is the next step in the intelligent automation journey. Organisations are solving data-driven machine learning use cases such as patient readmission, staff forecasting, medication adherence, and patient stay reduction and they are not stopping there. Instead, they are using the predictions to add new RPA automations that were not previously viable to solve more critical use cases, using multiple intelligent automation components together. Needless to say, it is an exciting time to be in this industry and driving real change for years to come.
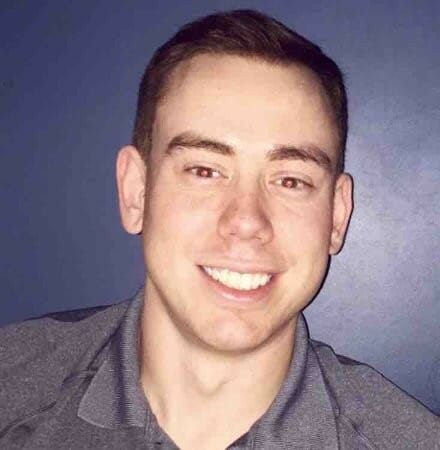