AI and machine learning have been driving significant transformations across virtually all industries over the last few years. By taking advantage of the technology, businesses can make sense of vast amounts of data to drive intelligent decision making and create new, innovative services to improve customer experiences. The banking sector in particular has benefited from AI and machine learning, especially when it comes to fighting fraud, which is a continuous, ever-changing threat that banks need to remain vigilant to.
At the same time, AI and machine learning powered technologies also ensure that security infrastructure doesn’t compromise on the customer experience. For example, consumers want to stay safe, without having to deal with unnecessary false positives or blocked transactions. Any friction points throughout the customer journey can cause frustration, and force customers into looking at competitors.
Here, we look at the different ways banks can take advantage of AI and machine learning to keep customers secure, while maintaining an exceptional customer experience.
Data analysis and threat detection
One of the main strengths of AI and machine learning algorithms is their ability to process vast amounts of data in real-time. The algorithms in use today take into account hundreds of factors, such as the device used, transaction history, the customer’s location and other contextual data to build up a detailed picture of each transaction and analyse the risk of that transaction within the context of the user and organisation.
Use cases for AI and ML in cyber security
As such, this risk-based approach is able to detect complex patterns in vast pools of structured and unstructured data, making AI-powered tools significantly quicker than humans at detecting new and emerging security threats, be that a spike in traffic from an unusual source, or a suspicious transaction that may require additional authentication.
For example, if a customer wants to check their bank balance from a recognised device and location, they would only need to go through basic authentication requirements to gain access to their account, such as entering a PIN. For higher-risk activity that fall outside of normal behaviour, such as an unusually large transaction amount in a new location, additional authentication will be required, for example a fingerprint or facial recognition.
Furthermore, because AI and machine learning algorithms are capable of analysing much larger data points, connections between entities and fraud patterns, the prevalence of false positives can be drastically reduced. This means fewer customers will be falsely rejected for fraud concerns, in turn minimising the labour and time costs associated with allocating staff to review flagged transactions.
So, by using AI, banks can analyse in real-time a wealth of information from several different data sources and channels, allowing them to make critical security decisions almost instantaneously and prevent fraud, without compromising on the customer experience.
Identity verification
Another example of how AI can be used by banks enhance both security and customer experience is with identity verification.
Banks have long relied on legacy, manual methods of verifying a customers’ identity, such as asking them to come into branch with their ID. As digital banking continues to boom, and data breaches expose more personal information across the web, it becomes more challenging to securely identify users without compromising the experience of the customer.
How to run a successful IT team in the banking sector
By combining legacy identity verification methods with AI and machine learning techniques, banks can achieve extensive, context-aware identity verification, preventing identity fraud and allowing customers to open bank accounts or take out new products without needing to come into branch. This includes a number of checks such as ID document capture, cross-referencing biometric data such as a selfie with an ID, device location, real-time account checking, and more.
Looking ahead
It’s clear that advances in AI and machine learning have been instrumental in enhancing security for consumers, enabling new services, and enhancing the user experience. However, we also know that AI and machine learning on its own will not be enough to prevent cyber attacks on the banking sector now, or in the future.
Cyber criminals are always on the look out for new vulnerabilities they can exploit that will give them the greatest return-on-investment. It’s no surprise to see attacks increase in both online and mobile channels, particularly as remote banking grows in popularity.
Banks and financial institutions need to be particularly vigilant when operating in new channels, or offering new products, that security is built in from the start and not added as an after-thought.
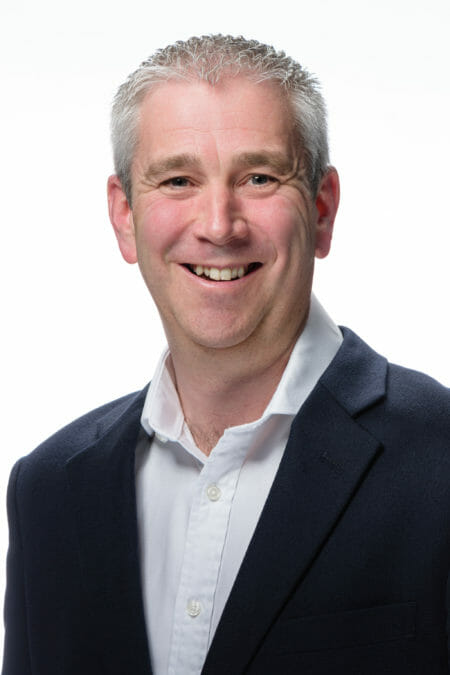