AIOps is quickly gaining prominence, with many IT leaders incorporating it into their digital strategies. In fact, Gartner estimates that 10% of organisations are already using AIOps as part of their business practices. This adoption is only set to continue as analysts predict more than 40% of businesses will adopt AIOps over the next two years. However, as with many technologies, the objective function of AIOps has been buried in a lack of understanding, confusion and hype. Where some IT teams are led by some vendors to expect too much or are aiming for too much too fast, other teams are still casting doubt on what AIOps really means, and underestimate its potential for their business.
So, we need to take a step back and understand what AIOps actually is, before we look to its use cases. Essentially, it is applying artificial intelligence (AI) and machine learning (ML) to enhance and automate IT operations. Historically rooted in IT Incident Management and incident correlation, this approach is quickly becoming an essential aspect of observability. It enables teams to not only correlate incidents but also surface unknown issues early and automatically, alert the right team immediately and provide precious root cause analysis, context and remediation runbooks – or even trigger automated remediation workflows.
When integrated with observability and the large amounts of real-time software telemetry it collects, AIOps has huge potential and can help prevent and solve a plethora of IT challenges. But, before jumping in headfirst, it’s important to separate the facts from the fiction to truly understand how AIOps can help enterprises thrive.
AIOps is just another meaningless term – true?
AIOps is much more than another buzzword or a simple tool to correlate incidents. When implemented properly, AIOps can detect anomalies automatically and help remediate and prevent incidents before they impact end-users and customers. Once anomalies or incidents are detected, it takes a further step and provides structured analysis and detail on what these issues are and what the root cause is. This allows the IT team to understand the problem within minutes, and fix it faster, preserving user experience and avoiding disruptions to the business.
This is observability in action. When working with telemetry data, AIOps can pick the right team to alert of issues it detects early, and provide actionable insights so that operations become more efficient and DevOps teams can focus on innovation, rather than spending non-productive time reactively troubleshooting problems.
Has ‘digital transformation’ become a meaningless buzzword?
AIOps only works for large organisations – true?
There is no real reason why a smaller teams could not use AIOps to differentiate their business and correct operational issues and also decrease human burden. In fact for small teams, AIOps can help to quickly discover issues and decrease pressure on already busy teams who need to eliminate toil to focus on value creation.
Actually, regardless of shape and size, all businesses can reap the benefits of AIOps. Smaller and larger teams will see quick results and effective outcomes from highly effective pre-trained ML models out-of-the-box, while larger teams will be able to fine-tune their models to best match their operational environments as they scale.
AIOps isn’t affordable and is too complex for our organisation – true?
Not true – there are various AIOps tools available with very different characteristics. While some tools indeed operate as opaque black-box systems providing little explainability and trust on their results, others do require considerable time, effort and resources before they can yield true benefits.
However, modern AIOps platforms have addressed these challenges. Benefiting from extensive existing telemetry and operational data sources, pre-trained ML models can immediately deliver solid and effective results without any long training periods and unaffordable costs. This makes getting results quicker, which in turn enables fine-tuning of applications and capabilities, based on company-specific data and rules.
AIOps is only effective in large projects – true?
Organisations are used to hearing about and seeing AI/ML fight and fix big problems, and so more often than not, organisations are very ambitious when it comes to the deployment of these technologies. This can lead to over-promising, under delivering and a lack of results. The same applies to AIOps. Many struggle to get projects across the line, and this ultimately leads to a waste of resources.
Tackling this issue when using AIOps means taking smaller steps, which eventually lead to bigger wins. Programmes that deliver tangible results more immediately will help build positive momentum. As teams learn and iterate in a shorter timeframe, they will quickly refine their approach, leading to more sustainable, beneficial strategies.
AI regulation for the EU: shot in the arm or shot in the dark?
AIOps will take my job – true?
It’s no secret that the idea of technologies taking over human jobs has been widely discussed in recent years. AIOps as a standalone tool doesn’t replace human work. It actually provides humans with augmentation, allowing IT teams to have more free time away from troubleshooting. In turn, this means human workers can focus on more valued, strategic work. Organisations that take on AIOps become more proactive and even preventive, delivering sttonger performance and productivity. Engineers end up doing less guesswork, building better software and more resilient systems. This all contributes to innovation, which ultimately leads to business growth.
We’re also still a little way away from AIOps functioning without the need for any human support. Today, we see AIOps primarily used to do the things we’ve talked about; detect problems, provide root causes, streamline resolution processes, and trigger downstream actions. This still requires human knowledge to define which workflows are and should be automated and which runbooks should be carried out.
Making fully autonomous decisions on any incident remains the next frontier for AIOps.
AIOps – revealing the truth
As we’ve seen, observability and telemetry software can really advance the use of AIOps. This enables tangible and immediate benefits to organisations through proactive anomaly detection, noise reduction, root cause analysis and incident workflow automation. While AIOps might not be able to tackle every single challenge DevOps teams face, it can improve observability capabilities, which is becoming ever more critical.
When businesses begin taking AIOps seriously and uncovering the truth of how it functions, we can look beyond the myths and use it as an innovative and analytic tool. Those that embrace this technology alongside AI/ML and observability will reap the benefits – and ultimately achieve or exceed their business goals.
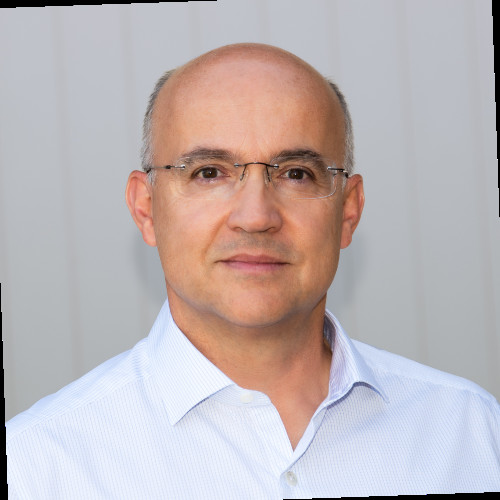