Dan Onions, global head of data management at Quantexa, explains how AI can help financial services institutions see the real world behind their data
One of the most pervasive problems in the financial services industry is managing the immense influx of data pouring into the systems. This data is meant to drive innovative growth strategies, help reduce risk and improve customer experience, but financial companies are held back from this purpose because they can’t see the data in context, so they miss how it fits into the real world that affects them.
Q&A: Dataiku VP discusses AI deployment in financial services
The data decision gap
Fragmented datasets across multiple internal systems have plagued companies for years, making it difficult for organisations to harness the full potential of their data. The recent Data in Context research study found that over 94% of financial services organisations suffer from a data decision gap — the inability to bring together internal and external data to support strategic and operational decisions. This gap throttles their abilities to act effectively with all the information at hand, and puts them at risk of regulatory compliance issues, missed customer experience opportunities, employee retention problems, and resource drainage due to heavy manual data workload.
Giving real-world meaning to data
Companies are working with an incomplete picture of the world when their datasets don’t represent real-world entities, relationships, and the context that binds them. For example, a financial services organisation often uses different systems for each of the products it offers to customers, with data not being joined together. When trying to make informed decisions about a given customer, financial services professionals need to take into account all the available data they have on that customer — the products they use and the systems they are on — to figure out the right course of action, but they can do so only if they are able to look at that data holistically, or ‘in a single view’.
The secret lies in optimising the data management process, but to do so manually requires an amount of effort many companies simply cannot afford or struggle with. Data can get old quickly, which means it takes sustained energy and constant monitoring to keep it up to date. Human teams, whatever their size or skill, simply cannot cope with it if they want to have the huge competitive advantage of real-time information. Instead, AI and automation can put companies back in control of their data.
How to approach modern data management and protection
Contextual Decision Intelligence to the rescue
Excellent data management rests on three principles: providing the right data, at the right time to the right people. Yet it becomes harder and harder to fulfil. It’s hard to find the right data when there is an ever-increasing volume and variety that has an impact on good decisions. The right time is elusive when data often needs to be real-time, but could be overwhelming if not properly aggregated and filtered into meaningful insights. The right people should be involved when there needs to be a human in the decision process so they are not snowed under with details that prevent them from doing their job, but are able to deep dive when needed. Artificial Intelligence is the key tool here, but it’s only as good as the data fed into it. When data is not joined up then results can be misleading and inaccurate, which often means data scientists spend more time trying to wrangle data and less time on the model effectiveness.
Seeing and understanding data in context is the deciding factor in surfacing hidden risks and opportunities. The cutting-edge approach available to financial services is known as Contextual Decision Intelligence (CDI), and it helps improve the data foundation, connect datasets wherever they are, and process them with AI and automation applications to help human teams make better-informed decisions.
Three core modules underpin CDI: entity resolution, network generation and advanced analytics. Entity resolution links internal and external data to better understand real-world entities and their behaviour – for example, internal company customer records joined with external data (such as corporate registries or watchlists). This generates a network, automatically unearthing sets of resolved entities and relevant links, creating a dynamic view of the picture. Finally, advanced analytics help to identify and score opportunities and risks through scorecards, statistical models, AI and machine learning to optimise automated decision-making. Meanwhile, human experts contribute to transparent contextual models by identifying patterns based on their experience. This allows decision-makers to visualise and explore data in a far more cohesive manner.
Simply put, the benefit AI brings to financial services organisations is eliminating the blind spots caused by poor data foundations, and leveraging the insights from huge volumes of internal and external data. In this way, AI enables organisations to harness the power of their own data instead of drowning in it, and with the CDI approach to scale the entire operation as the company grows and expands.
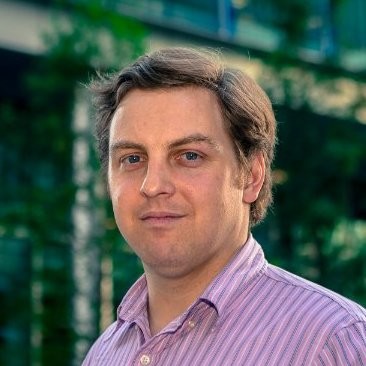