Atul Sharma, co-founder and CTO of Peak, discusses how decision intelligence is helping organisations unlock the potential of collected data
Data collection is skyrocketing. The amount of data created, consumed, and stored worldwide is set to increase by over 50% between now and 2025. Businesses understand evaluating their data more effectively provides a competitive edge, and that it will be artificial intelligence (AI), not BI that will unlock this potential — but there’s a striking gap between the scale of AI investment, and tangible returns delivered.
Fortune 500 companies are spending an average of $75 million on AI talent. But only 10% of all machine learning models are actually being put into production with an organisation. Now, a technology known as decision intelligence (DI) is helping companies bridge the gap between AI in theory, and productionising AI.
A commercial application of AI to the decision-making process, decision intelligence helps workers use AI insights in real time to make better decisions for their business — from choosing how to target ad spend, to reducing carbon emissions within supply chains.
With Gartner predicting that over a third of large organisations will be using DI within the next two years, now is an exciting time for businesses to explore potential inroads for adoption.
The nuts and bolts of decision intelligence
While AI can be a somewhat nebulous concept, decision intelligence is more concrete. That’s because DI is outcome-focused: a decision intelligence solution must deliver a tangible return on investment before it can be classified as DI.
A model for better stock management that gathers dust on a data scientist’s computer isn’t DI. A fully productionised model that enables a warehouse team to navigate the pick face efficiently and decisively, saving time and capital expense — that’s decision intelligence.
Since DI is outcome focused, it requires models to be built with an objective in mind and so addresses many of the pain points for businesses that are currently struggling to quantify value from their AI strategy. By working backwards from an objective, businesses can build needed solutions and unlock value from AI quicker.
Decision intelligence in action
Say a warehouse manager, let’s call her Sarah, is dealing with a stock shortage issue. Using the data she has available for the warehouse, Sarah bumps up orders across 200 stock-keeping units (SKUs). A month later, she’s grappling with yet another shortage.
But, with the help of DI, Sarah can make a decision that wouldn’t normally be available to an individual worker: one informed by extensive data overlaid with an intelligence that provides predictive insight. Taking into consideration circumstances across the business more broadly, Sarah’s DI platform recommends that she decrease orders from her supplier. Then, it has identified that her company has a warehouse with a depot one county over, with 2,000 units of that SKU that aren’t selling. It’s already alerted the logistics team, and has routed her scheduled delivery via that warehouse to pick up the additional SKUs. It will continue to run the same model to commercial teams across the business, adjusting the recommended action as data insights shift.
Meanwhile, department leads and executives have visibility into how their top-level decisions are impacting these smaller decisions at the process level. At a glance, they can see the challenges and advantages of different scenarios for different levels of the business, and make smarter decisions at pace and at scale.
DI adoption in global businesses
Global companies, including Pepsico, KFC and ASOS have already emerged as early adopters of DI, using it to increase profitability and sustainability, reduce capital requirements, and optimise business operations. As we continue to see more real-world case studies of DI in action, these are some of my favorite examples that showcase the power and potential of this technology:
Building greener, more efficient supply chains
Folks who work in the supply chain will be familiar with “stock vacations” — and the huge cost they entail for businesses.
It goes like this: distribution centre A is low on stock, so you shift product over from distribution centre B. Two weeks later, distribution centre B is low, so you have to shift it back again. Every time the stock moves, you’re paying for haulage and creating extra logistics work for busy teams, as well as generating an unnecessary carbon impact from the additional journeys.
Using DI, teams were able to optimise stock levels and allocations within a global CPG company, significantly reducing the movement of stock between distribution centres. The new efficiencies were made by analysing several factors, including demand (actual and forecasted), production output, processing costs, and transport costs, all of which helped to reduce truck journeys by 200,000 km — the equivalent of 147 tonnes in CO2 emissions — ultimately optimising operational costs and transport distribution from factory, to distribution centre, to supermarket.
Optimising warehouses to deliver a better customer experience
Warehouse logistics are intricate and complex. A tiny decision can be the difference between a package arriving on time with the customer, or getting significantly delayed.
Our team recently worked with a leading online fashion retailer to help ensure their next-day deliveries made it out the door on time. To make it happen, we had to find a manageable way to increase product picks per warehouse worker per minute.
We gathered all the relevant data and used DI to create a machine learning solution that predicted the optimal schedule of orders for each pick-wave. The outcome was a 5% increase in picks per person per minute, and a significant reduction in expedited logistics costs. It’s easy to see how the impact of this decision scales across the value chain, from the warehouse to customer experience.
A critical next step for AI
AI has been a buzzword for many years, but the more we look at it through the lens of real-world DI applications, the more tangible its potential.
Currently, 78% of businesses investing in data warehousing have yet to fully realise ROI. Decision intelligence can help those businesses get data out of silos and into the hands of commercial teams. It’s these teams that will deliver the value of data back into the business — and the wider world.
Especially when we consider the potential impact of AI-informed decisions on urgent global issues like sustainability, it’s clear there’s no time to waste in making the transformational potential of AI a reality with DI.
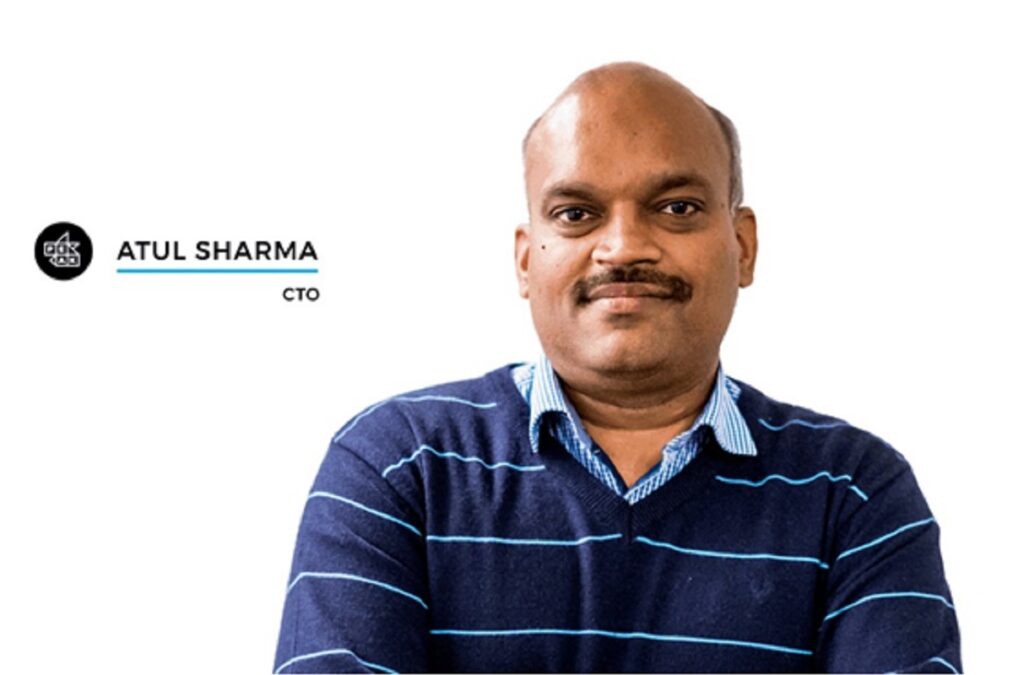
Related:
How data protection can benefit from artificial intelligence — This article will explore the ways in which data protection can benefit from artificial intelligence, as cyber attacks continue to grow and evolve.
Why AI is critical to seeing the real world behind financial services data — Dan Onions, global head of data management at Quantexa, explains how AI can help financial services institutions see the real world behind their data.