For a while now, the position of data scientist has been one of the most hyped roles in technology and, indeed, business. It’s not hard to see why – as organisations wake up to the seemingly limitless potential in their data, they’ve realised they need people that can extract, analyse and interpret large amounts of data. The demand is such that there is ongoing talk of a data scientist shortage, particularly in more experienced, senior roles.
Yet for all this attention, how effective are those data scientists, and how empowered do they actually feel? It’s a pertinent question, coming at a time when so much data is underutilised. Are businesses, knowing they need to make better use of their data, hiring data scientists without fully understanding how best to deploy the talent?
Perhaps a better way to look at it is to ask whether businesses know how to make better use of their data – are they hiring data scientists and expecting them to work miracles, or are businesses ensuring that not only do they have the right talent, but that they are feeding these teams with the right data?
How to embark on a data science career
Rubbish in, rubbish out
Many might think that it’s the job of the data scientist to find the right data, but they’re wrong. Ultimately, data scientists can only work with what they’re given, in the same way that a salesperson can only do so much with a poor product, or a Formula One driver can only achieve so much with an average car.
What, then, is the right data? Obviously, that varies from business to business, but fundamentally there are a number of principles that good data will follow, irrespective of organisational need. Firstly, it needs to be fresh – that means it needs to reflect the real world as it is at that moment. Everything changes so fast that a lot of data quickly becomes irrelevant. The more it stagnates, the less value it has.
So, if a data scientist is working on old data when there is more recent information available, the insights they can extract are going to be less relevant to the environment the business is operating in.
Secondly, it needs to be live data – so it needs to be from the real world, not training data, and not made up. Why? Because the real world is messy, throwing up anomalies that no one would ever have thought of, creating obstacles that models and indeed data scientists brought up on sanitised training data won’t be able to process.
Put another way – if an organisation feeds its data scientists and their models stale, offline data, then the best that enterprise can hope for is irrelevant, limited insights.
Why the edge is the next frontier for data scientists
That means businesses need to find a way of continually feeding their data scientists with live, evolutionary data, in real-time, from the real world. How do they do that? With edge computing.
Edge computing needs no introduction – with the explosion in Internet of Things devices over the last few years, more and more data processing is happening at the edge of networks. Sensors on everything from wind turbines and tractors to fridges and streetlamps are capturing data constantly. It’s real, it’s live, it’s messy, and it is exactly what data scientists need to be working on.
Businesses need to empower their data scientists by giving them training data and performance metrics from the edge. They can then use this to inform their AI models, which in turn are then deployed onto edge devices. These real-world environments give data scientists vital information on how their models stand up to anomalies and variations that can’t be recreated in labs or test environments. The models could well perform badly, at least initially – that’s a good thing, as it gives data scientists something to dig into, to understand what’s come up that they hadn’t thought of.
That said, whether the models perform well or poorly, data needs to be accessed, cleaned, annotated and ultimately fed back into the model for training on a continual basis. It’s a feedback loop that needs to keep running so that systems can improve and adapt. But it needs to be a smart extraction of data – no system can possibly manage all the data sensors are collecting, so having a way of identifying and getting the most important data back from the edge is critical.
On top of that, data scientists need to be able to redeploy sensors and machines to investigate, re-image and analyse data sources confusing the AI models. Whichever way the data has been gathered, however automated the process, at some point it was subject to human thinking, assumptions and presumptions. These may have been based on the data and evidence available at the time, but that may no longer be appropriate to capture the data needed. This is where being able to shift what data is being collected is vital for data scientists to remain effective, working on the most relevant information.
Training machine learning models to be future-ready
A new paradigm of active learning
Ultimately, this all signals a shift away from the old paradigm of collecting big sets of training data, segmenting, training the model and seeing what happens, and towards a new paradigm — one of active learning, where AI models learn how to cope with the real world, and data scientists are empowered to work effectively. In doing so, they will be better equipped to gather the insights and intelligence needed to give their organisations a true competitive edge in increasingly crowded, data-driven marketplaces.
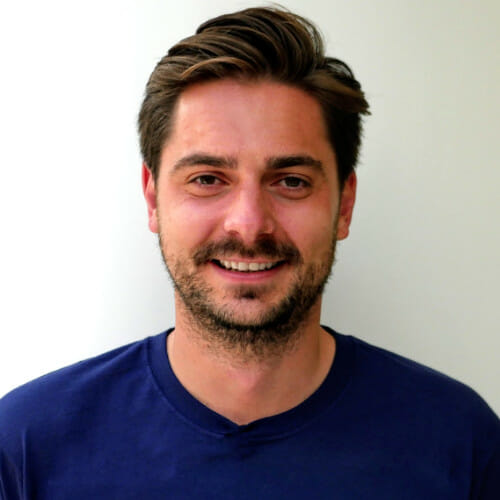