Marco Gallieri, senior researcher at NNAISENSE, discusses how digital twins can boost electric vehicle (EV) battery life and performance
Electric vehicles are taking over the car market – and it’s happening faster than most people realise, according to experts. But key to that growth — and for individual EV makers to distinguish themselves in an increasingly crowded market — is better battery technology. When EVs become more widespread, those with more efficient or longer-lasting batteries will stand out.
In addition to concerns about efficiency, batteries include metals and resources that significantly fluctuate in price, and suffer from supply chain challenges; and, there are serious environmental impact issues in the manufacture, maintenance, and disposal of electric vehicle batteries. Thus getting the most out of a battery — ensuring it lasts as long as possible and performs as well as possible throughout its lifecycle — is a paramount objective for EV makers. And one of the most efficient ways manufacturers can accomplish this is by applying artificial intelligence to train digital twin (DT) models for battery manufacturing, testing, and maintenance.
This is not just a theory; DT technology has been used in real-life EV and HEV battery development and management, including in some Formula One race cars, where I was part of a project to develop DTs that resulted in superior battery management for a hybrid single-seater. In fact, DTs are “a leading technology” in Formula One racing, according to a top UK digital twin research centre.
How digital twins work
Digital twins are digital representations of an object – in this case an EV battery – that can be analysed to understand how the battery will react in real-world situations. The DT model embeds knowledge about the battery’s characteristics — size, shape, range, materials; its ability to interact with other systems, e.g. a HEV energy recovery system; as well as its current physical state. The model can thus discern likely behaviour under specific circumstances. The DT is developed using advanced artificial intelligence, including machine learning and neural networks, to accurately model the process dynamics, based entirely on sensor data. Advanced AI is the central element in the next generation of the digital twin.
Using the information from the digital twin model, manufacturers can determine the exact and most efficient balance for one of their biggest challenges — maximising range and power while ensuring as long a life for the EV battery as possible. Although manufacturers today offer warranties on their batteries for as long as eight years — guaranteeing customers they will not see a significant drop in performance for that period — the issue of EV battery degradation is a real one. Speed; distance driven; time between charges; quick charges; and even weather can affect the life of a battery. Oversizing the battery is often the go-to solution to ensure that the battery performs for the term of the guarantee. But, this redundancy is expensive, and releases extra waste into the environment.
Increasing efficiency and sustainability
While manufacturers are hard at work developing solutions for battery efficiency, digital twin technology — especially as it has advanced since the Formula One community first embraced it nearly a decade ago — can help them more easily resolve some thorny dilemmas. For example, efficiency in battery charging requires the delivery and integration of chemicals and components in the charging system. Achieving the ideal balance that will enable the most efficient charging is critical, and requires a clear understanding of the performance envelope; an imbalance could not only harm battery life and efficiency, but even cause an explosion. DT technology enables researchers to deploy the most effective techniques, and to validate their results on the most advanced data-driven simulations, ultimately achieving the ideal balance between components and the charging system before deployment. This ensures that batteries charge as efficiently as possible, with the results delivered in as efficient a manner as possible.
The same technology model can be applied to many other aspects of battery manufacture and use — for example, analysing components used in battery manufacture to determine the most environmentally-friendly materials; producing the best charging schedule to ensure the longest possible battery life; developing the most effective techniques to renew deteriorated batteries; and finding the cheapest, most effective, and most environmentally-friendly way to dispose of batteries whose lifecycle is at an end.
Manufacturers, who need to source components and deal with regulatory issues (especially regarding end of life, disposal, and recharging depleted batteries) are not the only ones who benefit from DT technology; it can also help resale value for owners, who on average keep new vehicles between five and eight years before selling. Although the used EV market is still small, it will grow significantly as EVs become more common. Thus, battery longevity is likely to be a major factor in the value of a used EV. DT technology in battery production and management can thus ensure that batteries are running at optimal levels when it’s time to trade an EV in, with owners getting the most they can for their vehicles, and buyers of used EVs assured that they are making a good purchase.
While EVs don’t emit fossil fuel pollutants like internal combustion engine-equipped vehicles do, they still have their own sustainability issues, including their reliance on certain mined minerals and the disposal of used batteries. And a battery that does not operate as efficiently as possible could harm a vehicle’s performance and value. If batteries last longer and work better, they will need to be charged, recycled or disposed of less frequently— better for manufacturers, and for drivers. Digital twin technology has the ability to accomplish that. If it’s good enough for Formula One, it’s good enough for the rest of us, too.
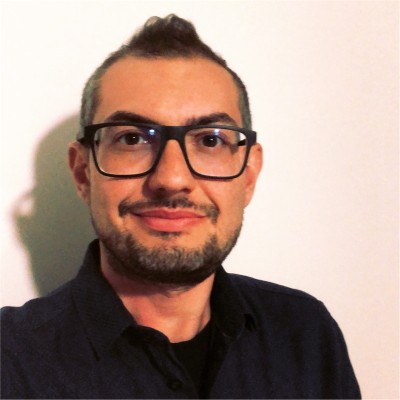
Related:
Why do digital twins need the edge? — Craig Beddis, CEO and co-founder of Hadean, explains why digital twins need the edge in order to truly prosper.
The value of real-time and historic data in manufacturing — Thomas Degen, solutions engineer industries at KX, discusses how combining real-time and historic data can improve the manufacturing process.